Cell-ebrating gene diversity!
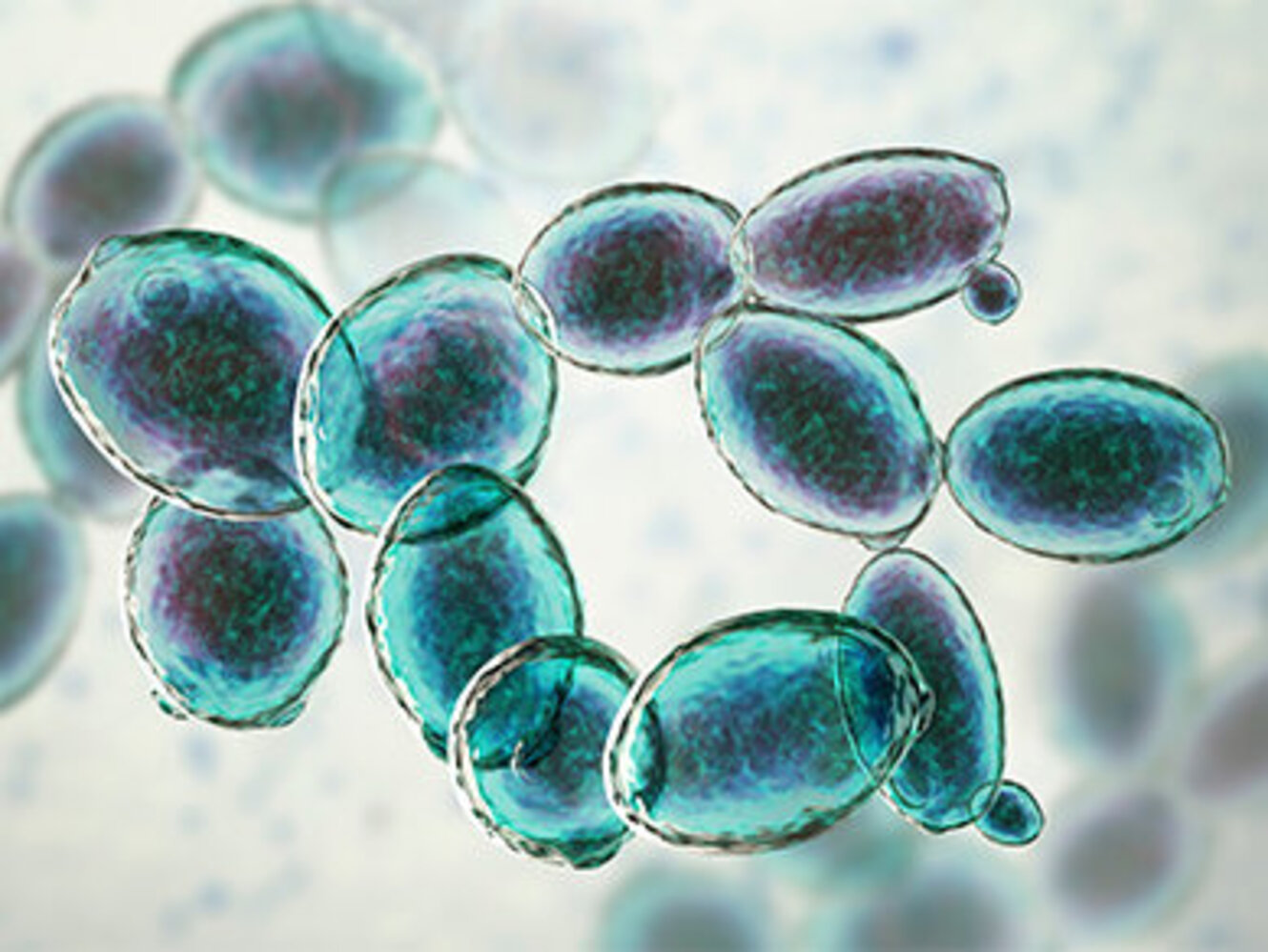
The precise regulation of the yeast Saccharomyces cerevisiae cell cycle relies heavily on the orchestrated expression of cyclins, which activate CDK1 (cyclin-dependent kinase 1). While many cell cycle-related genes exist as paralogous pairs, they are often assumed to have redundant functions and similar expression patterns. The Theoretical Biophysics Group (Edda Klipp) challenged that notion by employing single-molecule inexpensive fluorescence in situ hybridization (smiFISH) to meticulously analyze the expression of five key paralogous gene pairs across numerous unsynchronized yeast cells. Utilizing a convolutional neural network (CNN) for precise cell cycle phase classification, they revealed significant differences in expression levels, correlation, and temporal profiles among these paralogues. Furthermore, they explored the compensatory mechanisms between paralogues upon gene deletion a functional divergence of cell cycle-related paralogues, suggesting they play distinct roles in the intricate regulation of yeast cell cycle progression. Check out their Cells Article!
Abstract
Cell cycle progression of the yeast Saccharomyces cerevisiae is largely driven by the expression of cyclins, which in turn bind the cyclin-dependent kinase CDK1 providing specificity. Due to the duplication of the yeast genome during evolution, most of the cyclins are present as a pair of paralogues, which are considered to have similar functions and periods of expression. Here, we use single molecule inexpensive fluorescence in situ hybridization (smiFISH) to measure the expression of five pairs of paralogous genes relevant for cell cycle progression (CLN1/CLN2, CLB5/CLB6, CLB3/CLB4, CLB1/CLB2 and ACE2/SWI5) in a large number of unsynchronized single cells representing all cell cycle phases. We systematically compare their expression patterns and strengths. In addition, we also analyze the effect of the knockout of one part of each pair on the expression of the other gene. In order to classify cells into specific cell cycle phases, we developed a convolutional neural network (CNN). We find that the expression levels of some cell-cycle related paralogues differ in their correlation, with CLN1 and CLN2 showing strong correlation and CLB3 and CLB4 showing weakest correlation. The temporal profiles of some pairs also differ. Upon deletion of their paralogue, CLB1 and CLB2 seem to compensate for the expression of the other gene, while this was not observed for ACE2/SWI5. Interestingly, CLB1 and CLB2 also seem to share work between mother and bud in the G2 phase, where CLB2 is primarily expressed in the bud and CLB1 in the mother. Taken together, our results suggest that paralogues related to yeast cell cycle progression should not be considered as the same but differ both in their expression strength and timing as well in their precise role in cell cycle regulation.